Author: Volnyanskyi A.
For several years now, unbeknownst to ourselves, we regularly encounter machine learning, one of the technologies that is a field within artificial intelligence (AI). It is thanks to this technology that we receive relevant responses from the assistant on our smartphones, and content recommendations from services like Netflix and YouTube. We explore other circumstances under which we might encounter machine learning in our daily lives.
- Text translation
- Optimizing Email Management
- Choosing the Best Route and Predicting Travel Time
- Necessary Purchases
- Tagging Friends in Photos on Social Networks
- Customer Support Services
- Medical Diagnostics
Text translation
GNMT by Google (Google’s Neural Machine Translation) is one of the most famous practical examples of using machine learning. This system contains tens of thousands of dictionaries and uses natural language processing to improve translation accuracy. While previously Google Translate only recognized text, today it translates entire web pages, documents, and even text in images on the fly.
Optimizing Email Management
And again, it’s Google, this time with its Gmail application, whose efficiency in spam sorting reaches 99.9%! Using special filters, Gmail identifies potential spam based on the sender’s address, place of sending, subject, and other parameters of the email. The rest of the emails, including those from unknown senders, are sorted into folders such as “Promotions,” “Social,” “Updates,” and “Forums.”
The following types of machine learning are recognized: supervised, unsupervised, semi-supervised, reinforcement, and deep learning. The last option, deep learning, is capable of producing results at the output that are comparable to the performance of the human brain.
Choosing the Best Route and Predicting Travel Time
Uber users often don’t realize that machine learning is used to calculate travel time. Implementing this technology significantly improved the accuracy of travel time predictions by 26%. Additionally, the taxi booking app uses historical data based on previous trips in the area—both yours and those of other users. By the way, Uber’s dynamic pricing is also determined using machine learning, specifically through a model called Geosurge.
According to Statista, the machine learning market is expected to grow at an annual rate of 18.73% and reach $528.1 billion by 2030. A significant portion of the market is in the USA.
Colobridge’s Expert:
“Artificial intelligence is already changing conventional work approaches in our field of activity. For example, a typical data center can provide clients with computing resources based on their actual needs, resulting in uneven loading of physical equipment while cooling systems operate at the same performance in all machine rooms. Solutions using machine learning help optimize energy consumption in real-time—thanks to this, heavily loaded equipment will be cooled more intensively and vice versa.”
Necessary Purchases
Marketers can be blamed for making us buy more than we planned. On the other hand, thanks to the use of machine learning, many shopping platforms often offer products that we really need. Such recommendation systems take into account the user’s preferences, location, gender characteristics, and previous shopping experiences to provide accurate recommendations.
Tagging Friends in Photos on Social Networks
About ten years ago, Facebook developed DeepFace—a facial recognition project based on deep learning (one of the types of machine learning), which identifies human faces in digital images, including those far from portrait quality. Pose, facial expression, and even lighting hardly affect the accuracy of recognition. Thanks to this, the popular social network is highly likely to “recognize” people and suggest tagging them in the photos you upload.
Customer Support Services
Live operators, at least on the first line, have been replaced by chatbots and virtual agents. They are always online, respond instantaneously, and their performance is not affected by fatigue or personal issues. Machine learning in their case is used for speech recognition, converting it into text and vice versa. One major bank assessed the accuracy of such a chatbot and found that it provided correct answers in 96% of cases! At the same time, the highest level of acceptance of these “smart assistants” is observed in the e-commerce segment.
Medical Diagnostics
Machine learning is already being used to analyze radiological studies (for example, during lung cancer screening), to find fractures and tumors that are difficult to detect with the human eye, and to develop new treatment protocols based on historical data in the form of patient charts. Pfizer, which created one of the most famous COVID-19 vaccines, for example, uses IBM’s Watson machine learning to develop immuno-oncology drugs.
According to Statista, in which industries is machine learning most commonly used :
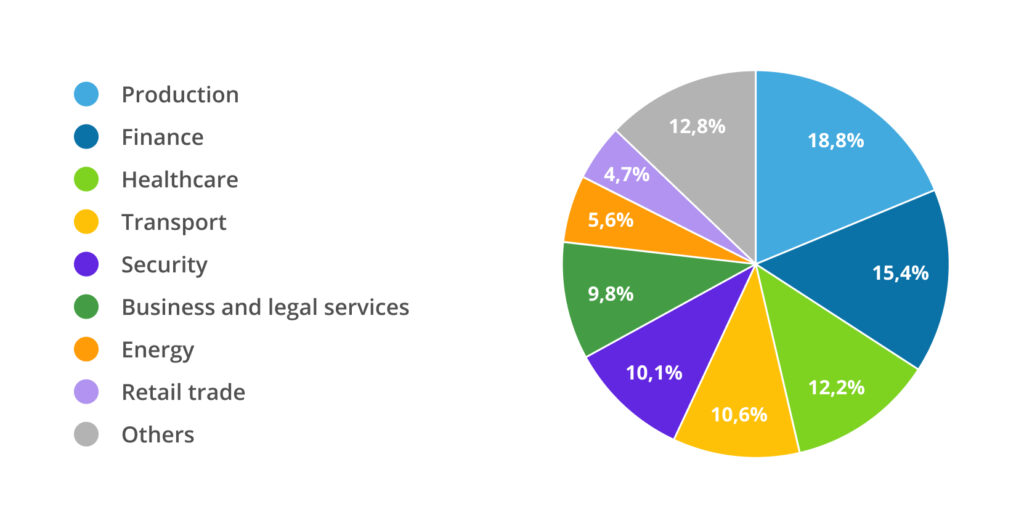
If we talk about the tasks that can be solved using machine learning in business, these primarily include quality analysis of historical data for making forecasts, creating recommendation systems to retain customers and increase revenues, detecting fraud cases, efficient planning, automating routine operations, and many others.
Colobridge’s Expert:
“For companies that have large volumes of data about their clients, as part of our new project, we offer AI-Engine as a Service — a multifunctional high-performance solution for real-time data analysis. Accurate analysis and forecasting tools help businesses make more informed decisions, increase operational efficiency, manage risks effectively, and stay competitive.”
For more information on how to use machine learning and other artificial intelligence methods, please consult with the managers of Colobridge.