Author: Volnyanskyi A
Predictive analytics is already being used to assess demand for goods and services, determine fraud risks, calculate the probability of equipment failures, and personalize marketing offers. In other words, it’s a powerful tool that brings benefits to various fields of activity. Learn more about what predictive analytics is, how it works, and what other opportunities it opens up for businesses and beyond in our article.
- Definition of Predictive Analytics
- How does predictive analytics work?
- Three Predictive Analytics techniques(Forecasting Models)
- Uses and examples of predictive analytics
- Top Predictive Analytics Tools
Definition of Predictive Analytics
Predictive analytics is one of the areas of data analysis focused on forecasting future events based on historical data. Knowing with high probability what will happen has become possible thanks to the development of artificial intelligence, machine learning, and statistics. Together, they are used to identify patterns and determine likely scenarios for event development with high accuracy.
According to Fortune’s estimates, the global predictive analytics market, which was $18.02 billion as of the beginning of 2024, is projected to grow to $95.3 billion by 2023. This will be driven by increasing interest in AI-based forecasting and the number of cases of implementation such solutions, further penetration of technologies into all sectors of the economy, and the integration of predictive analytics with artificial intelligence and IoT.
How does predictive analytics work?
Data specialists addressing various tasks requiring precise forecasting, days, and months ahead typically employ similar approaches in their work. Roughly, the entire process of obtaining a forecast can be divided into five stages
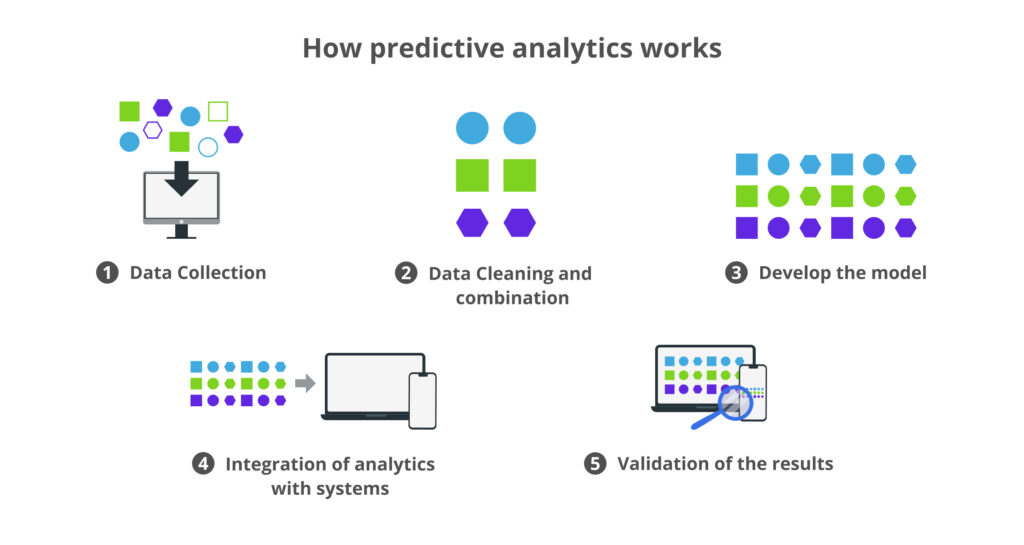
There’s another slightly different sequence of steps to achieve the same results:
Stage 1: Task Definition. Requirements for forecasting results are formulated. For example, assessing the probability of loan default in a bank or determining inventory sizes before “Black Friday” so that all interested customers can purchase items from the website.
Stage 2: Data Preparation. Data is collected and organized by the organization. Historical data accumulated over several years as well as current data generated through customer interactions are required. It’s convenient to work with data stored in specialized repositories.
Stage 3: Data Preprocessing. Data is cleansed to remove anomalies and data obtained under atypical conditions for the organization to reduce the likelihood of forecasting errors.
Stage 4: Development of Predictive Models. The most suitable model is chosen depending on the task formulated in the first stage and the available dataset. Machine learning is often used, although other options are possible, such as regression models or decision trees.
Stage 5: Results Verification. The accuracy of the predictive model is tested, and adjustments are made until it starts producing acceptable results.
Three Predictive Analytics techniques(Forecasting Models)
Let’s revisit the stage of developing forecasting models and take a closer look at the methods used in predictive analytics.
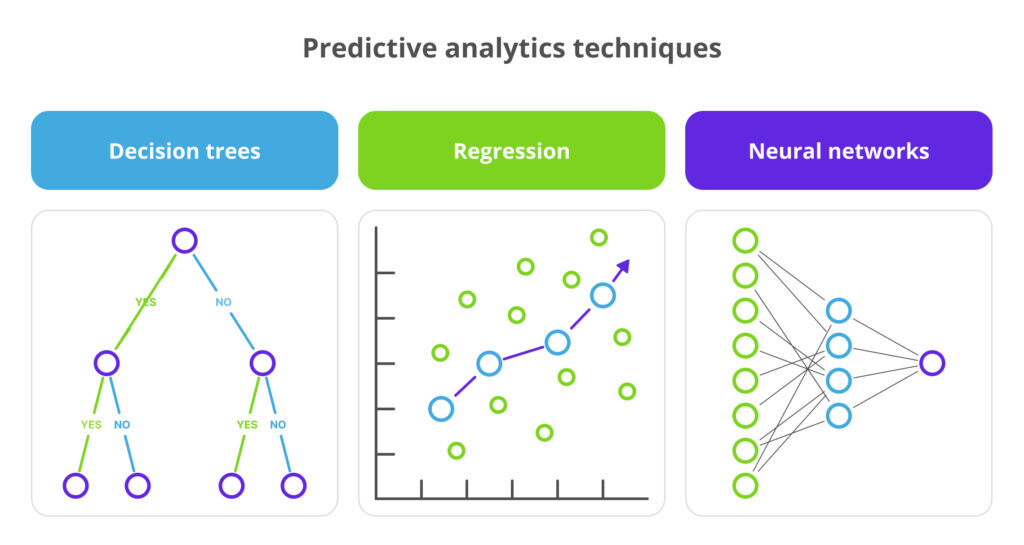
Decision Trees
Decision trees are used to visualize and analyse potential scenarios in conditions of uncertainty. They attract attention with their clarity, making it easy to represent even the most complex scenarios in an understandable form while simultaneously considering all possible outcomes. To obtain results, a tree with nodes—decision points—is constructed, and then for each node, a question or condition for transitioning to the next node is formulated, after which the probabilities and values of each scenario are summed up.
As an example, let’s consider the operation of a typical store. A decision tree will help evaluate the location of the future point of sale and select the assortment of goods considering optimal location and target audience.
Regression Analysis
Using Regression Analysis to Analyse Relationships Between Variables
Regression analysis is utilized to assess relationships between variables, particularly to uncover patterns within large sets of continuous data. It can involve predicting qualitative indicators, assessing forecast accuracy, or examining the influence of one variable on another. This method is prized for its high precision as well as its simplicity in both application and interpretation.
In our example, regression analysis will enable us to determine how factors such as product cost, marketing effectiveness, and seasonality impact sales volumes.
Machine Learning (Neural Networks)
An optimal choice for complex modelling, specifically in uncovering neural connections (patterns that are imperceptible to humans) within large datasets. It is renowned for its accuracy, efficiency, and scalability. Suitable for cases where standard mathematical formulae cannot be applied to solve a problem, automating data analysis processes, and building precise predictive models. Algorithms can be trained on datasets with either known or unknown outcomes, as well as through trial and error in a simulated environment. Neural networks can be used in conjunction with two other models for verifying their results.
Machine learning can be highly beneficial in: personalizing marketing offers, forecasting demand within specific time frames, assessing fraud risks, and predicting customer churn probabilities.
Uses and examples of predictive analytics
The examples provided above with the offline store are just one of the many ways to harness the potential of predictive analytics. Today, it is applied in marketing, finance, healthcare, manufacturing, retail, telecommunications, logistics, energy, and the public sector.
Predictive analytics capabilities:
- Forecasting: Predicting demand for goods and services, customer behaviour, organizational financial indicators, risks (customer churn, equipment failure, etc.).
- Segmentation: Segmenting customers based on their life cycle, behavioural factors, and demographic characteristics.
- Creating personalized offers: Recommendations (as done by YouTube, Netflix) and marketing content.
- Optimizing: Pricing, marketing campaigns, business processes.
- Detecting anomalies: Fraud, equipment failures, data discrepancies.
- Visualizing: Results of predictive analytics, identifying patterns in data.
Predictive analytics can thus be beneficial in various tasks and fields of activity.
Colobridge’s Expert:
“Combining predictive analytics and cloud technologies enables quick and efficient processing of large volumes of data using cutting-edge forecasting methods. Moreover, thanks to the cloud (specifically, virtual infrastructures), predictive analytics becomes more accessible to companies that previously didn’t utilize it due to lack of expertise and corresponding IT infrastructure. In the cloud, a platform for predictive analytics can be deployed fairly quickly and easily scaled if needs change over time: data volumes increase, new objectives arise, etc. Essentially, cloud technologies have already become a game-changer for predictive analytics—altering significantly how decisions based on data are made.”
Top Predictive Analytics Tools
When it comes to predictive analytics, the following tools are commonly used:
- Python: A programming language with a rich set of libraries for machine learning and data processing. Suitable for most tasks in predictive analytics.
- R: A programming language specifically designed for statistical modeling. Often used for data processing and analysis.
- SAS: Software suite for data processing, business analytics, and forecasting. It enables discovering patterns in data and visualizing results. Applied in risk analysis, demand forecasting, and customer segmentation.
- Power BI: Microsoft’s software solution for business analytics and data visualization with forecasting capabilities. Well-suited for simple forecasts, trend analysis, and data visualization.
- Microsoft Excel: A popular application within the MS Office suite supporting statistical analysis tools (regression). Due to limited functionality, it’s used for trend analysis and basic forecasting.
If you’re unsure how to get started with predictive analytics or what resources and IT skills are needed, we’re here to help. Reach out to Colobridge specialists, and we’ll offer the best predictive analytics solution tailored to your needs, using cutting-edge methods and technologies.