Author: Volnyanskyi A.
Let’s continue discussing how to improve customer communications through a Data-Driven approach. This article focuses on extended segmentation (microsegmentation): it significantly enhances the accuracy of customer relationships and leads to outstanding results.
- Traditional Segmentation vs. Microsegmentation
- Technological Underpinnings of Microsegmentation
- What’s Next?
Traditional Segmentation vs. Microsegmentation
Traditional segmentation allows for the identification of broad customer groups based on generalized characteristics. This primarily includes demographic data, past purchasing behavior, and product preferences. Microsegmentation, on the other hand, is a more detailed approach. It divides customers into small segments with a detailed set of criteria, taking into account numerous factors: from company social media activity to transaction history and customer reviews. Thanks to this, it’s now possible to identify segments such as “customers aged 30-35 who order home delivery in gift packaging several times a year” or “customers aged 20-25 leading an active lifestyle, preferring lactose-free products, and paying for orders online.”
Thus, microsegmentation not only divides customers into obvious categories but also takes into account a large number of factors: demographic, historical, behavioral, transactional, customer lifecycle forecast, and associated risks. All this is done to consider their preferences during brand communication and understand why a particular offer would appeal to them.
Microsegmentation has become possible thanks to the use of analytics. Descriptive analytics allows for the assessment of current behavior, while predictive analytics predicts future actions. The real value of microsegmentation for businesses lies in its ability to create highly accurate offers for customers, thereby increasing engagement, satisfaction, and loyalty while simultaneously reducing churn and rejection rates. This enables companies to flexibly adapt marketing strategies to narrow customer segments, promptly respond to changes in their preferences, and thereby gain additional competitive advantage.
Technological Underpinnings of Microsegmentation
At the core of microsegmentation are machine learning algorithms that analyze historical data to identify patterns, forecast future behavior, and perform diagnostic analytics to understand the reasons behind these patterns. Importantly, the process of segmenting into microsegments is not static but dynamic, and the results become increasingly precise as new data emerges. Analytics tools, leveraging artificial intelligence, are utilized for data processing. This enables the discovery of hidden (not obvious to humans) patterns and correlations.
Colobridge’s Expert:
“The Colobridge technological platform was utilized to deploy a machine learning model tailored to the needs of a cosmetics company. The company aimed to provide higher-quality customer service and enhance interaction with their clientele. Traditional segmentation approaches proved inefficient, and there was no clear understanding of which among the vast amount of data was truly valuable for the business. Colobridge, in collaboration with its subsidiary Beinf, employed factual, predictive, and diagnostic customer characteristics, integrating them with predictive analytics. This allowed for a comprehensive understanding of each customer microsegment: identifying their real needs, preferences, and assessing future outcomes from communication. This information was subsequently utilized for marketing communications with customers. The computational power required for this task was provided by Colobridge, eliminating the need for the client to build complex infrastructure and hire staff for maintenance and support.”
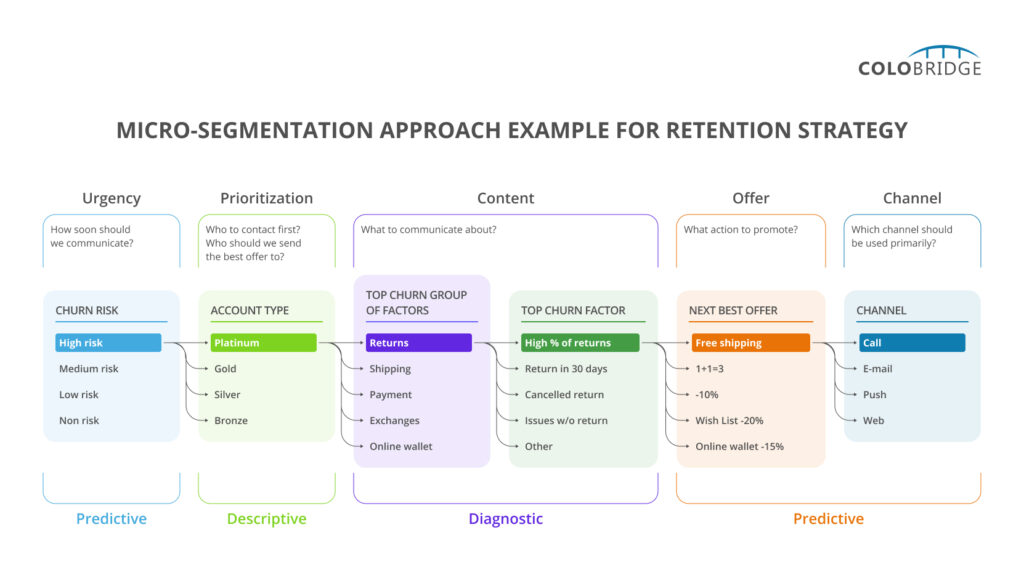
The image above depicts one of the microsegmentation methods developed for customer retention. Historical data attributes and forecasts of potential events were utilized for this purpose. Among these attributes were account type, churn risks, optimal communication channels for delivering personalized offers, and others.
All these attributes are united by their real business value in the context of solving a specific task.
For example:
- Churn risk: the higher it is, the more precise and “aggressive” the communication should be.
- Account type: it impacts revenue and engagement, allowing identification of customers who potentially bring in more profit.
- Communication channel: it affects the likelihood of response and the expected impact.
Based on these and other attributes, a microsegment of customers can be identified. For instance, customers with a Platinum status who, due to a high rate of returned items, may switch to competitors. In this case, the most effective strategy would be a personalized call from a manager offering to deliver the next order for free.
What’s Next?
After microsegmenting the customer base, the next step should be personalizing messages. In the next session, we will explore how to automate this process to relieve the marketing department from the routine task of preparing dozens, hundreds, or even thousands of individualized offers.
Discover at Colobridge how to leverage artificial intelligence, machine learning, and predictive analytics to increase business profitability. We will not only provide the technical foundation for implementing your projects but also offer all the necessary expertise.