Author: Volnyanskyi A.
Continuing our coverage of improving customer relationships using the Data-Driven CRM Framework—our approach to systematizing customer relationship management. We explain what data management is and why it is the foundation for building an effective data-driven marketing strategy.
- Customer Relationship Management and Data Quality
- Data Engineering: Transforming Raw Data
- Data Storage Systems: Ensuring Data Integrity and Availability
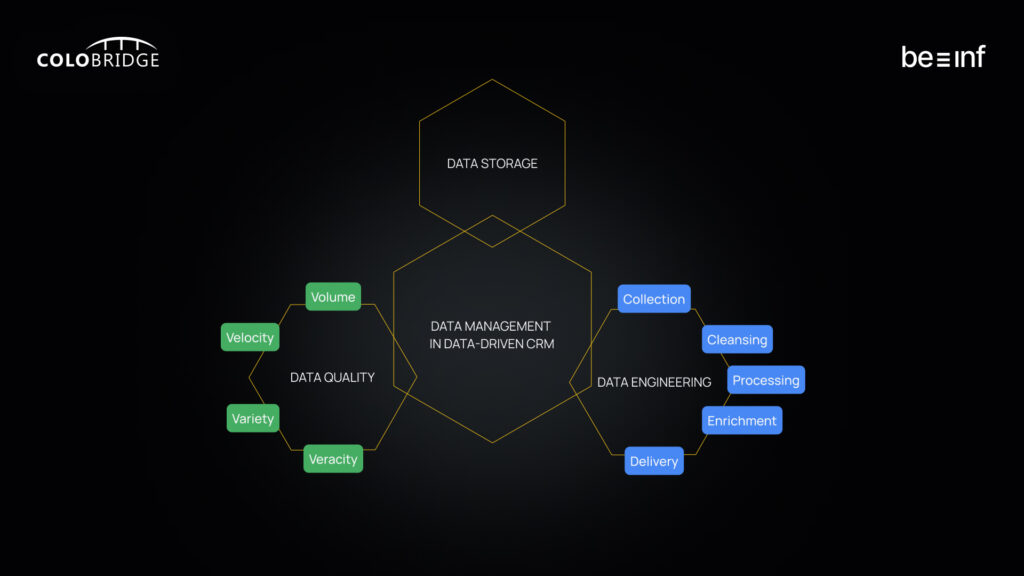
As an example, let’s take the case of our Beinf project for an online store specializing in fashionable clothing, shoes, and accessories. This company aimed to improve the shopping experience while maintaining high-quality service, which required the implementation of new approaches to interacting with store customers.
Customer Relationship Management and Data Quality
Quality data is the driving force behind the entire customer relationship management mechanism. Without data that meets certain conditions, even advanced analytical tools and strategies won’t work effectively.
Ideally, data should meet the four Vs:
Volume: Ensure that you have enough data.
Example: Significant data volume is required to make informed decisions. For example, a complete list of transactions, history of interactions with customers, and their feedback on products over several years. These and other data will provide a reliable basis for subsequent analysis, unlike data sets that only cover the last few months.
Velocity: Assess the speed of data creation and movement.
Example: Ideally, important data are collected and processed in real-time. The faster data is generated and processed, the greater its impact on decision-making.
Variety: Use a diverse range of data that comprehensively characterizes the user.
Example: For quality analytics, it’s necessary to track all aspects of customer interaction with the product/system: product views, additions to favorites, adding to the cart without completing a purchase, and others.
Veracity: Consider the uncertainty of data, paying special attention to its reliability and origin.
Example: The platform collects data from various sources, and their accuracy may vary. To ensure consistency and reduce the risks of erroneous data affecting decision-making, it is necessary to compare new data with existing data. It also makes sense to merge data from different systems—for example, linking data related to purchases on an e-commerce website with its mobile application.
Colobridge’s Expert:
“Data quality is not just the driving force behind the effective implementation of customer engagement strategies; it is the actual fuel of your business. It is thanks to this that you will be able to effectively implement strategies in marketing and sales, achieving impressive results.”
Meeting the four Vs is just the beginning. To fully benefit from data that meets these criteria, the data itself must be properly prepared. We explain how to do this.
Data Engineering: Transforming Raw Data
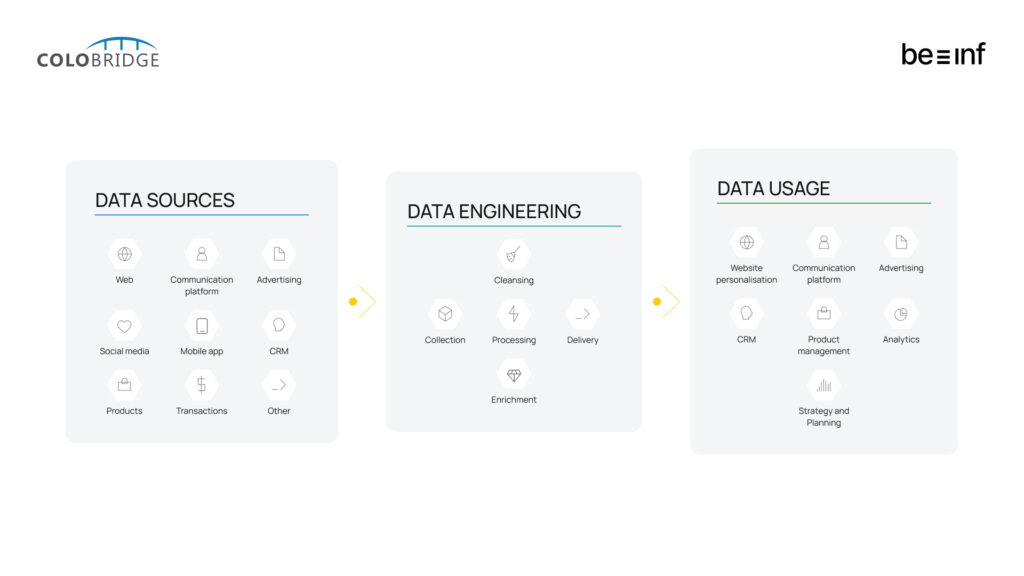
To make raw data suitable for further processing, it must sequentially go through each of the stages described below.
- Data collection from various sources according to set goals.
Example: An online store for clothing, shoes, and accessories collects data on customer behavior on its website, including information about viewed and cart-added items, and completed purchases. Data also comes from social networks and external sources (demographic data, customer preferences).
- Cleaning, which includes correcting errors and removing incorrect/inaccurate data.
Example: During data analysis, the company discovered that some records had spelling errors in the names of clients, and certain fields with contact information were missing. With the use of data cleaning software, typos are corrected and empty fields are filled to ensure the necessary accuracy and completeness of data.
- Processing, which includes organizing, tabulating, and compiling the cleaned data.
Example: In processing transactions, the company calculated the total payments for each client over a certain period and calculated the average check. For further analysis, transactions were classified by types: purchase of goods, payment for services, and others.
- Enrichment by adding additional variables for deeper analysis.
Example: A set of attributes was developed that comprehensively characterizes the customer according to several parameters. For example, whether they have children, pets, or a car, whether they shop only for themselves or for all family members, and what style of clothing they prefer.
- Delivery, which involves making data easily usable by end-users or systems.
Example: The company developed an interface for its employees where they can easily access necessary information about the customer (purchases and other actions) for more effective interaction with them.
Colobridge’s Expert:
“You can use the above examples as a brief guide to obtaining quality data for further analytics and making informed decisions. It is precisely this that ultimately allowed the company from our example (a marketplace for clothing, shoes, and accessories) to make informed decisions and reach a new level of communication with customers. A similar approach can be applied in other business areas as well.”
Significant resources are required for collecting, storing data, and processing it. Let’s consider the criteria they must meet.
Data Storage Systems: Ensuring Data Integrity and Availability
To address these two tasks, effective data storage needs to be organized, which can be implemented in various ways. The two most popular are Customer Data Platforms (CDP) and Data Warehouses (DWH):
Customer Data Platform (CDP)
This is a system that collects customer data in real-time from all sources. This approach allows for a unified customer database that is accessible to other systems. CDPs are suitable for companies that prefer to work with data as it accumulates, need a unified representation of data from various sources, and ultimately plan to use a personalized approach in marketing. To implement a CDP, it is necessary to assess your data sources (interactions with the website, CRM system, social networks, etc.) and choose a service provider who can seamlessly integrate them with the platform. Examples of CDPs include Segment, Bloomreach Engagement, Totango, Blueshift, Insider, Listrak, Lytics, and Treasure Data.
Thanks to the integration of different types of data (structured and unstructured, obtained from online and offline sources), CDPs help improve customer service quality (CX). CDPs create a continuously updated comprehensive view of the customer based on data collected from all communication channels—email, social networks, loyalty programs, and in-store operations—as well as data stored in other internal systems.
Data Warehouse (DWH)
This is a large centralized database optimized for analytics and reporting, operating on the principle of batch processing. It is suitable for storing, processing, and analyzing large volumes of data.
DWH is used by companies that require advanced analytics, reporting, and analysis of historical data. Data warehouses are well-suited to handle large volumes of information and execute complex queries. To start working with a DWH, it is necessary to develop a schema that meets the analytical needs of the company and consider factors such as data granularity, indexing, and partitioning. Cloud platforms are best suited for deploying a DWH. Popular examples of cloud data warehouses include Amazon Redshift, Google BigQuery, and Azure.
A data warehouse extracts information from multiple sources, including relational databases and transactional systems, and can be connected to business analytics tools that help gather, analyze, visualize, and create reports on the data. DWH operates with static data, ensuring their integrity and instant access, and is best suited for historical and financial analysis.
The choice between CDP and DWH for a medium-sized business depends on its goals and requirements. If real-time data handling and personalized marketing are priorities, CDP is suitable. If comprehensive analytics capabilities and reporting creation are needed, DWH is a more appropriate option.
Consult with Colobridge experts to assess data quality, determine the best solution for data storage and processing, and learn how to use data to improve your performance in marketing and sales.