Author: Bohomiakov V.
Artificial Intelligence (AI) is no longer science fiction—it has become a real-world tool that is transforming business processes and presenting company leaders with complex challenges. For CEOs and IT directors, implementing AI is not just an opportunity to boost competitiveness but also a necessity to address challenges related to data, financial investments, and change management.
AI and Data-Driven approaches are key concepts in today’s world, where technology defines success. How can businesses determine if they’re ready for AI? And what does preparing a company for a Data-Driven, AI-powered business—where data and technology work in synergy—look like?
McKinsey suggests that Data-Driven companies extract greater value from their data and achieve progress faster than competitors. Meanwhile, 60% of IT directors surveyed by Deloitte consider AI a critical factor for business success
- Key AI Challenges for CEOs and IT
- How AI Helps Businesses: Practical Examples
- How to Prepare a Company for AI: A Step-by-Step Plan
- Success Metrics and Return on Investment
- Conclusions
Key AI Challenges for CEOs and IT
The adoption of AI comes with obstacles and new challenges for CEOs and IT departments, as the final outcome depends not only on technical solutions but also on realistic expectations, resource management approaches, and other factors.
Understanding AI’s Role in Business
For many executives, artificial intelligence remains an abstract concept, and its potential is often underestimated. AI is not a magic button that solves all problems—it is a tool that requires clearly defined goals and objectives. Businesses may miss growth opportunities if they limit themselves to basic tools like chatbots without realizing how AI and a Data-Driven approach can tackle more complex tasks—from analyzing customer behavior to optimizing supply chains or forecasting demand.
The main challenge for CEOs and IT leaders is striking a balance between inflated expectations and the actual capabilities of the technology—ensuring AI integration becomes part of a broader Data-Driven business strategy.
Data Quality and Accessibility
AI can only function with high-quality, structured data. Companies that store information across disparate systems, on paper, or in outdated databases face the challenge of digitizing, consolidating, and cleaning it. Without this step, any attempt to implement AI and Data-Driven business practices is doomed to fail.
The IT department must build an infrastructure for data collection, storage, and processing, which demands significant resources, time, and expertise. For instance, if customer data is fragmented or lacks key events, AI predictive models will fail to deliver accurate forecasts—eroding trust in the technology.
Financial Investment and Scalability
Integrating AI into business is a costly endeavor, with expenses varying based on company size and project scope. Small businesses can opt for SaaS solutions with monthly subscriptions costing a few hundred dollars, making AI and Data-Driven approaches more accessible.
However, custom AI development for large enterprises—such as predictive models or large language models (LLMs)—can range from tens to hundreds of thousands of dollars. Even a successful pilot project may fall short if scalability isn’t planned: transitioning from testing to full-scale deployment requires additional investments in infrastructure, employee training, and process adaptation. This is especially critical for Data-Driven businesses, where data volumes grow exponentially.
Talent Shortage
Implementing AI in business requires skilled professionals: data scientists, machine learning engineers, cybersecurity specialists, AI architects, and other experts. However, the market faces a severe shortage due to high demand and fierce competition. Building an in-house team demands strategic planning, investment in training, and talent retention programs.
Executives must choose between outsourcing and developing internal capabilities, adding complexity to managing AI and Data-Driven business projects. A lack of expertise can lead to poor technology choices or misinterpreted results, undermining the effectiveness of AI initiatives.
Ethical and Legal Considerations
AI raises critical concerns around privacy, ethics, and regulatory compliance. For instance, while call analysis technologies can reduce fraud, they also risk violating data privacy.
Companies must navigate not only local laws but also international regulations if they serve global customers—adding legal complexity. For AI and Data-Driven businesses, this means implementing strict security policies and involving legal experts to avoid fines, lawsuits, or reputational damage.
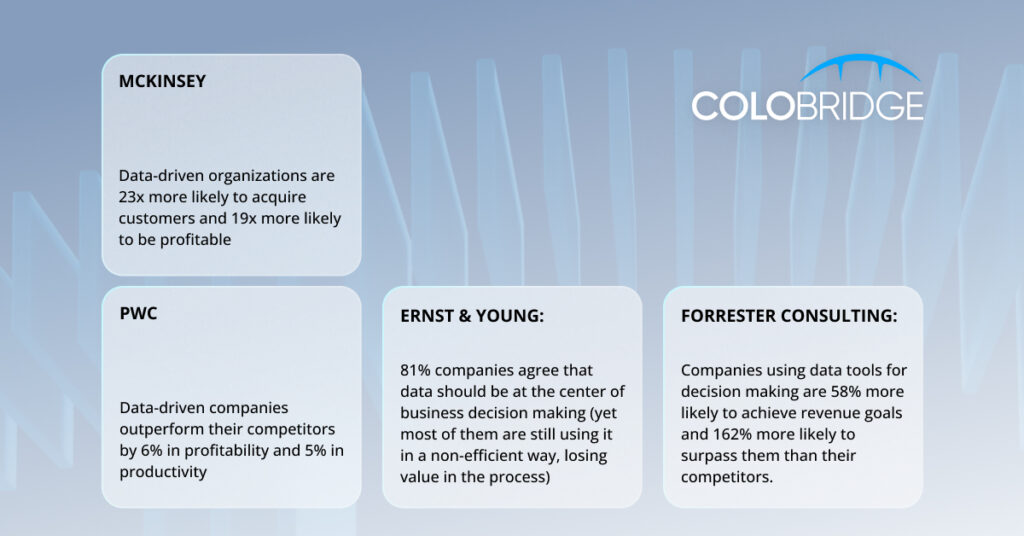
How AI Helps Businesses: Practical Examples
AI is transforming businesses—from sales forecasting to process optimization. Here are real-world use cases.
Predictive Analytics & Personalization
AI can predict customer behavior with high accuracy, helping businesses boost sales and retain audiences. For example, predictive AI can estimate the likelihood of a purchase within the next week and trigger a personalized offer based on the customer’s past behavior.
This is particularly valuable in retail, media, and B2C sectors, where an AI and Data-Driven approach reduces costs on mass marketing campaigns while improving efficiency. Businesses can focus only on customers who need a slight “nudge” to convert—avoiding wasted efforts.
Automated Content Generation
Generative AI assists in creating texts, scripts, visuals, and even customer service call scripts. Companies can instantly produce personalized emails tailored to specific audience segments or generate call center scripts—cutting preparation time significantly.
In industries like video production, AI already reduces reliance on junior staff, though human oversight by experienced professionals is still needed for quality control. Here, AI and Data-Driven business acts as a tool for streamlining creative workflows.
Process Optimization
AI in business significantly accelerates operational processes, making them more efficient. In the energy sector, for example, this technology reduces customer request response times from days to hours by analyzing inquiries and offering ready-made solutions using large language models. In manufacturing, AI optimizes product formulations, cutting costs and speeding up the development of new goods.
Other Opportunities
AI also aids in supply chain management, quality control, and even education. For instance, in logistics, it predicts supply chain disruptions; in the food industry, it optimizes production processes, minimizing defects; and in education, generative AI accelerates material preparation, saving instructors time. These cases highlight the versatility of AI and data-driven business, which can adapt to any industry when given a clear objective.
How to Prepare a Company for AI: A Step-by-Step Plan
We explain how to implement AI and a Data-driven approach in business in seven steps.
Step 1: Assessing Business Readiness
Before adopting AI, it’s crucial to evaluate how prepared the company is to transition to an AI and Data-driven business model. Key indicators of maturity include:
- Decisions in the company are made based on data, not intuition, reflecting a Data-driven approach;
- There is a centralized data storage system with fast access and a high-quality structure;
- Some business processes are already automated;
- Specialists are appointed to oversee the implementation and management of technologies (including AI). If data is still stored on paper, the first task is to digitize it and integrate it into a unified system, laying the foundation for an AI and Data-driven business.
Step 2: Setting Priorities
AI is effective only when there’s a specific problem to solve, such as customer churn, high production costs, or slow processes. A clear goal helps select the right technology—predictive models for forecasting or generative AI for content creation—and avoids unnecessary expenses. For example, if the business is losing customers, focus on predictive models; if marketing needs scaling, opt for generative solutions. This aligns with the Data-driven concept, where priorities are dictated by data.
Step 3: Testing Through a Pilot Project
A pilot project (Proof of Concept, PoC) allows you to test a hypothesis on a small dataset. For example, to analyze customer churn, a model is trained on historical data and tested on a sample where the outcome is already known. If the prediction accuracy reaches the target threshold (e.g., 85% or higher), you can plan the next step. Overall, a PoC minimizes risks and assesses the potential of AI and a Data-driven business for a specific task.
Step 4: Choosing an Implementation Approach
There are several options, each varying significantly in cost and complexity.
- SaaS solutions are suitable for small businesses with minimal infrastructure needs, starting at €300 per month.
- Custom projects are preferable for large companies with unique challenges and complex IT systems, with costs starting at €10,000.
- In-house team is a long-term investment for those who see AI as a core part of their Data-driven business strategy. This option involves substantial costs, often in the tens of thousands of dollars. The choice depends on the company’s resources, IT strategy, and readiness for change.
Step 5: Risk Management
To avoid failure, several factors must be considered:
- Data quality: A lack of necessary data or poor data quality can hinder the project.
- Scaling: Moving from testing to production requires resources and planning.
- Security: Data leaks through third-party tools pose a real threat to AI and Data-driven businesses.
- Expectations: Overly high hopes for AI can lead to disappointment without a realistic assessment.
Step 6: Investing in Talent
For success, you need specialists who can configure, maintain, and advance AI systems. Outsourcing is suitable for the initial stages, but in the long term, it’s beneficial for a company to build internal expertise. Training employees, allocating 10% of their work time to learning new technologies, and hiring tech leads with experience in AI and Data-driven business are essential parts of the process. Without this, even the best solutions will remain underutilized.
Step 7: Continuous Development
AI technologies evolve rapidly, and companies must be prepared to regularly update their models. For instance, large language models require retraining to prevent degradation and hallucinations. A Data-driven business implies an ongoing search for and adaptation to new tools, as well as allocating additional resources for experimentation.
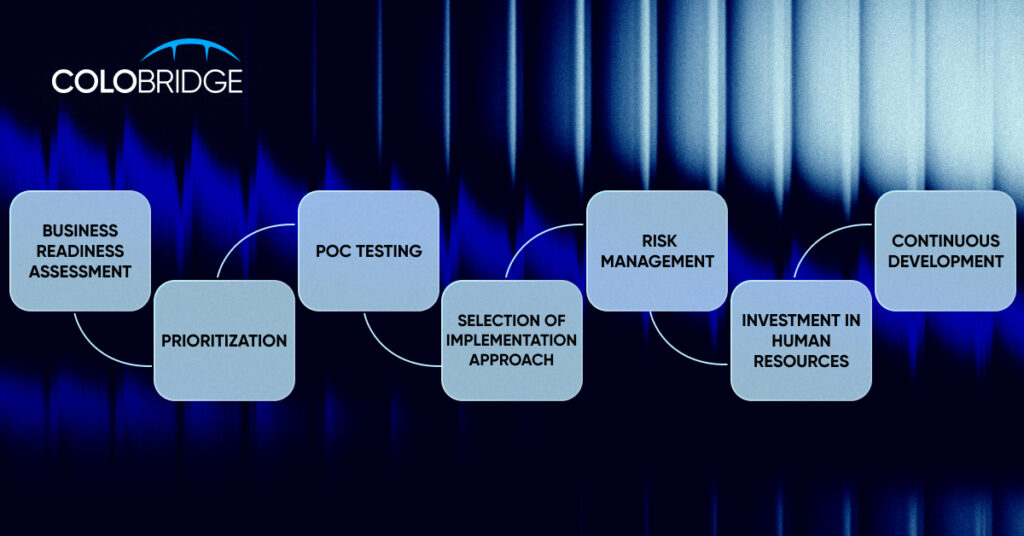
Success Metrics and Return on Investment
Evaluating AI effectiveness depends on the company’s goals. For sales, it’s about revenue growth or an increase in average order value; for automation, it’s about reducing time or costs; for customer retention, it’s the accuracy of predictions (e.g., 65-85%, depending on the task). Testing during the PoC phase allows you to measure results and determine whether the investment pays off. The key is to align the benefits with a specific business objective, whether it’s resource savings, as in production optimization, or additional revenue from personalized offers. AI and a Data-driven business deliver measurable results only when goals are set correctly.
Conclusions
Artificial intelligence is not a threat but a powerful tool that can elevate a business to new heights with the right approach. For CEOs and IT directors, it poses challenges related to data, costs, talent, and ethics, but it also unlocks opportunities for growth, optimization, and competitive advantage. Regardless of the business’s scale, success hinges on realistic expectations, high-quality data, and effective risk management. The question is not whether to implement AI, but how to do it in a way that makes it a driver of development and maximizes benefits in the era of thriving Data-driven business.